PARSISS supports and promotes a broad range of clinical demands in different surgical application domains. There are brief descriptions of the ongoing research projects.
Ongoing projects
Design and implementation of a neurosurgery planning platform based on artificial intelligence (AI)
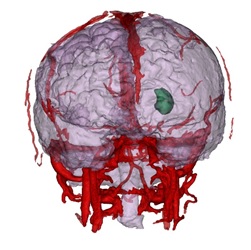
Neurosurgery planning is a time-consuming and complex task that requires a detailed understanding of the patient’s anatomy and the affected regions in the brain for neurosurgeons to precisely provide the treatment and avoid proximity to any known risky situations and critical structures. Conventional neurosurgery planning approaches are heavily dependent on the surgeon’s expertise and experience, which directly affects the surgical outcome. To improve surgical planning, neurosurgeons require accurate 3D visualization of critical structures such as tumors and vascular structures.
This study aims to design a platform for accurate pre-operative planning through the creation of patient-specific 3D models of vascular structure and brain tumors based on 2D MRI and evaluate its effectiveness via integration with navigation systems. As a result of using the proposed platform, time of planning, surgeon errors, and damage to vital structures will be reduced, and the outcome of neurosurgical interventions will be improved as a result of minimally invasive surgery.
The objectives of our research are summarized as follows:
Aim 1: Development of optimized AI-based segmentation methods for brain tumors and vascular structures segmentation problems.
Aim 2: To design an interactive tool for fine-tuning the results of AI-based segmentation by surgeons.
Aim 3: To improve a neurosurgical planning procedure by utilizing 3D visualization of patient-specific anatomical structures.
Aim 4: To assess the neurosurgery procedure by incorporating patient-specific 3D anatomical structures into the navigation system.
2.Design and implementation of the patient-specific 3D model of the liver and its structures
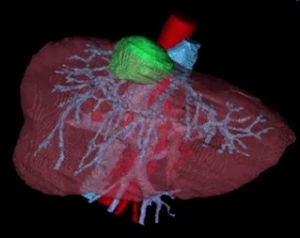
Liver surgery, especially live donor liver transplantation, is an important treatment option and standard of care for many forms of liver disease, such as acute liver failure, chronic liver disease, liver cancer, and congenital metabolic disorders. Despite significant improvements in clinical care, operative strategies, and technology over the past decades, liver surgery is still challenging and risky due to complex vascular structures such as hepatic and portal veins, and complex anatomy in different parts of the liver that change in each patient. Therefore, optimal pre-operative planning and anatomical assessment are necessary to minimize the risks of surgery.
This study aims to design and implement a patient-specific 3D model of the liver and its structures for improving a planning procedure through the registration of pre-operative critical structures that are extracted from CT and MRI data of patients. The objectives of our research are summarized as follows:
Aim 1: To develop accurate methods for vascular structures and tumor segmentation in pre-operative CT images.
Aim 2: To develop an accurate duct segmentation method in pre-operative MRI images of patients.
Aim 3: AI-based registration of extracted structures from CT and MRI.
Aim 4: To improve a liver surgery planning procedure by utilizing the patient-specific 3D model of the liver.
3.Automatic touchless registration of preoperative images and intraoperative coordination of a patient in navigation systems using a laser scanner
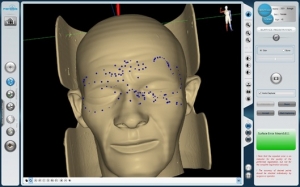
In navigation systems, registration of intraoperative patient coordination to preoperative images is performed using two conventional approaches of using a few anatomical landmarks and point clouds in patient space through contact with the surface of the body. Selecting and using anatomical landmarks largely depends on the user and needs more skill. It is while, access to a high-precision, non-contact point cloud could increase patient comfort, accuracy and speed of data acquisition, and reduce contamination. A points cloud could be created by moving an instrument over the patient’s body surface. Different approaches such as laser scanners, 3D scanners, and LED masks with known advantages for each of them are proposed to acquire accurate point clouds.
This study aims to design and implement an automatic touchless registration platform using a laser scanner to improve registration accuracy in navigation procedures. The objectives of our research are summarized as follows:
Aim 1: To develop a touchless registration setup using a laser scanner.
Aim 2: To reduce the registration process time in navigation systems.
Aim 3: To reduce the dependency of registration accuracy on an experienced user.
Aim 4: Automatic registration of preoperative images and intraoperative coordination of the patient based on AI algorithms.
4.Navigated Transthoracic needle aspiration (TTNA)
Transthoracic needle aspiration (TTNA) is a non-bronchoscopic approach to peripheral lung nodules that lie outside the airway (about 40% of nodules). Potential limitations of the procedure are inherent to the dynamic nature of the respiratory system. Therefore, the TTNA procedure can be done under the guidance of ultrasound, fluoroscopy, and CT imaging. However, each of these modalities has been proven to have well-known limitations. CT-guided TTNA as the most common image-guided procedure for TTNA has a good visualization capability. CT-guided TTNA faces the important challenge of high radiation doses to patients and surgeons during the procedure. Fluoroscopy-guided TTNA (F-TTNA) has been an alternative to CT-TTNA due to shorter durations, lower exposure, and cost. However, it suffers from poor visualization of small lesions. Ultrasound-guided TTNA biopsy provides non-ionized and real-time visualization, but with low contrast images. Navigated TTNA is a novel technology that provides the current position of the needle for the accurate localization of peripheral pulmonary nodules.
This study aims to design and implement a setup based on an optical navigation system to track the needle and respiratory motion during the TTNA procedures.
The objectives of our research are summarized as follows:
Aim 1: To develop a setup for an accurate navigated TTNA procedure.
Aim 2: To estimate and compensate the patient’s respiratory motion during the TTNA procedure.
Aim 3: Automatic registration of intra-operative data and pre-operative images based on AI algorithms.